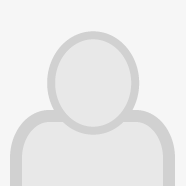
mgr inż. Milena Sobotka
Zatrudnienie
- PhD Student w Politechnika Gdańska
- Specjalista inżynieryjno-techniczny w Katedra Inżynierii Biomedycznej
Obszary badawcze
Kontakt dla biznesu
- Lokalizacja
- Al. Zwycięstwa 27, 80-219 Gdańsk
- Telefon
- +48 58 348 62 62
- biznes@pg.edu.pl
Media społecznościowe
Kontakt
- milena.sobotka@pg.edu.pl
Specjalista inżynieryjno-techniczny
- Miejsce pracy
-
Budynek A Elektroniki
pokój EA 207 otwiera się w nowej karcie - Telefon
- +48 58 347 17 35
- milena.sobotka@pg.edu.pl
Wybrane publikacje
-
Mask Detection and Classification in Thermal Face Images
Face masks are recommended to reduce the transmission of many viruses, especially SARS-CoV-2. Therefore, the automatic detection of whether there is a mask on the face, what type of mask is worn, and how it is worn is an important research topic. In this work, the use of thermal imaging was considered to analyze the possibility of detecting (localizing) a mask on the face, as well as to check whether it is possible to classify...
-
Impact of Visual Image Quality on Lymphocyte Detection Using YOLOv5 and RetinaNet Algorithms
Lymphocytes, a type of leukocytes, play a vital role in the immune system. The precise quantification, spatial arrangement and phenotypic characterization of lymphocytes within haematological or histopathological images can serve as a diagnostic indicator of a particular lesion. Artificial neural networks, employed for the detection of lymphocytes, not only can provide support to the work of histopathologists but also enable better...
-
Preeclampsia Risk Prediction Using Machine Learning Methods Trained on Synthetic Data
This paper describes a research study that investigates the use of machine learning algorithms on synthetic data to classify the risk of developing preeclampsia by pregnant women. Synthetic datasets were generated based on parameter distributions from three real patient studies. Four models were compared: XGBoost, Support Vector Machine (SVM), Random Forest, and Explainable Boosting Machines (EBM). The study found that the XGBoost...
wyświetlono 690 razy