Description
Many deep learning applications require figure-ground segmentation. The performance of segmentation models varies across modalities and acquisition settings.
The release of SegSperm dataset intends to contribute to the development of algorithms for:
(1) segmentation of small objects,
(2) segmentation of elongated objects,
(3) segmentation of blurred objects,
(4) Computer-Assisted Sperm Analysis (CASA) systems.
As embryologists seek high-quality sperm with desired shape and motion attributes to increase chances of fertilization, sperm assessment can take advantage of the segmentation task. Despite the remarkable progress of deep neural networks in figure-ground segmentation in the last decade, blurry microscopic images of minuscule sperm, with spatially uneven contrast and light variations, continue to challenge modern deep neural networks.
Most works prioritize efforts toward detecting sperm heads to simplify the problem at the expense of accuracy. Notably, the tail has also been shown to play a key role in assessing sperm quality. On the other hand, the segmentation of the flagellum is much more challenging than the segmentation of the head due to the microscopic video characteristics. The low field depth of microscopes blurs the images, easily confusing human raters in discerning minuscule sperm from large backgrounds during image labeling. To spur further progress on training full sperm segmentation algorithms with noisy and imbalanced ground truth labels, we release SegSperm - a new dataset of microscopic images of sperm.
The SegSperm dataset contains microscopic images of full sperm. The images were selected from videos of Intracytoplasmic Sperm Injection (ICSI) procedures. The dataset consists of 551 gray images of resolution 512×512 pixels and associated binary ground truth masks of full sperm. The training set consists of 432 images from 40 videos and the test set consists of 119 images from 9 videos. The binary masks of spermatozoa were segmented manually by one rater. In addition, 23 images of sperm from the validation set were annotated by two more raters. The ground truth masks of each rater were split into head and tail part.
If you use the SegSperm dataset in your research, please cite the following paper:
Lewandowska, Emilia, Daniel Węsierski, Magdalena Mazur-Milecka, Joanna Liss, and Anna Jezierska. "Ensembling noisy segmentation masks of blurred sperm images." Computers in Biology and Medicine (2023): 107520.
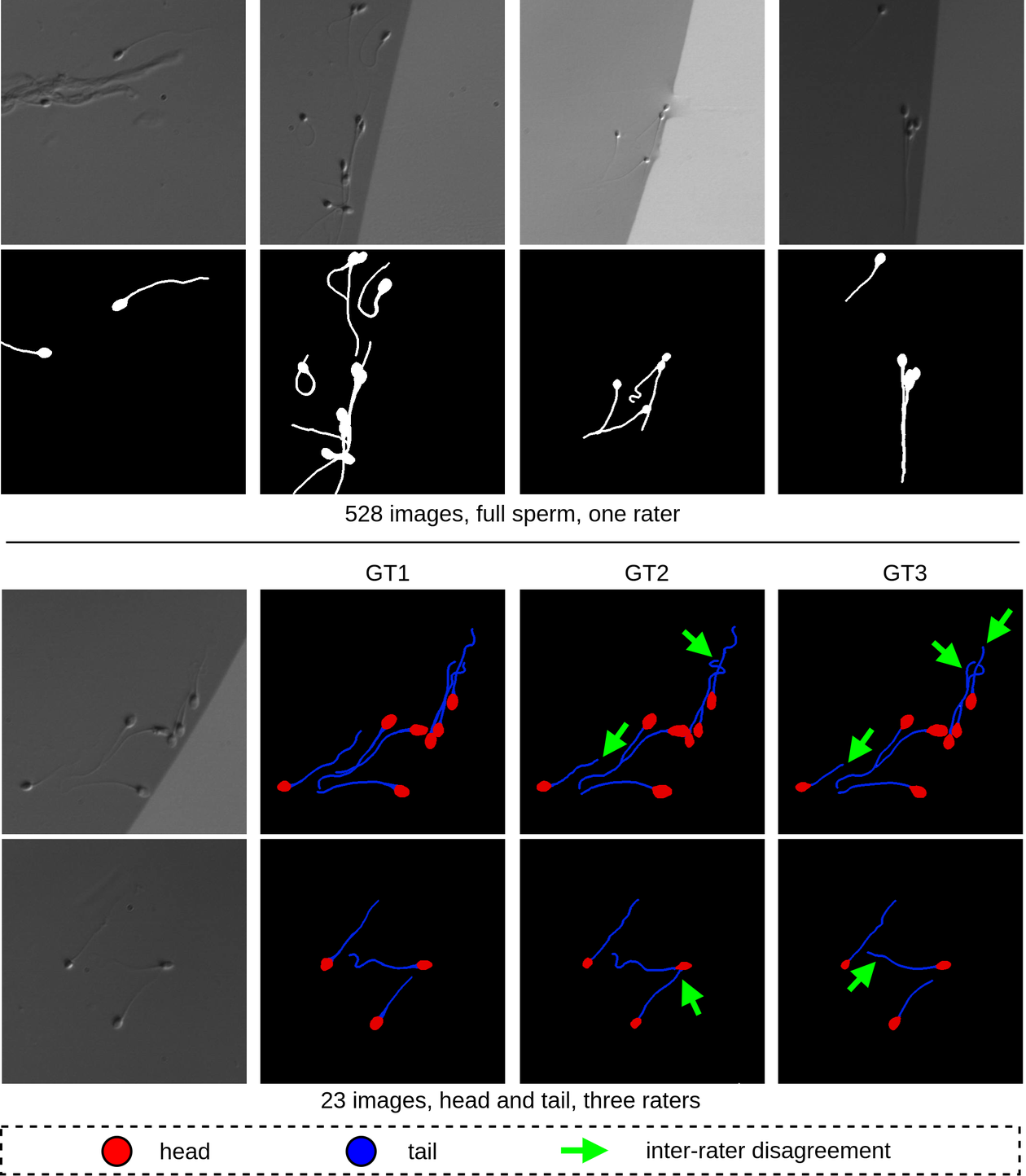
Dataset file
hexmd5(md5(part1)+md5(part2)+...)-{parts_count}
where a single part of the file is 512 MB in size.Example script for calculation:
https://github.com/antespi/s3md5
File details
- License:
-
open in new tab
CC BY-NCNon-commercial
Details
- Year of publication:
- 2023
- Verification date:
- 2023-02-28
- Dataset language:
- English
- Fields of science:
-
- information and communication technology (Engineering and Technology)
- DOI:
- DOI ID 10.34808/6wm7-1159 open in new tab
- Verified by:
- Gdańsk University of Technology
Keywords
- diagnostics
- microscopy
- sperm
- segmentation
- artifacts
- multiple labels
- in vitro
- deep learning
- blur
- small objects
Cite as
Authors
seen 719 times