Description
A dataset represents simulated images of depth sensor seeing a single human pose, performing 200,000 random gestures. The depth images as vectors of pixels are stored with ground truth positions of every relevant joint.
3D graphics software (Blender 3D) was used to model, pose and render human figure, creating depth image and appropriate ground truth for each pose. It was assumed, that the sensor has a horizontal field of view 57° (same as Kinect), and the depth image was rendered to 60×50 pixels in 8-bit greyscale (3-bit less, i.e. 8 times lower depth resolution than Kinect).
The human figure was positioned in an empty space, without a background and other foreground objects. In real applications to obtain the same conditions a separation of foreground object based on depth information should be performed, e.g. by assuming the body to be the object closest to a camera, and discarding the ones positioned further by comparing their depth values.
The dataset represents a wide variation of the upper body poses with biologically correct random joints positions, with wrists positions uniformly covering the available space in front of the figure. The body absolute position was changed randomly to introduce shifts in x, y and z directions relative to the fixed sensor. The movement ranges were limited to allow only far reaching hands to leave the frame, whilst the rest of the figure remained visible. 200,000 depth images were created.
The applications of the database include training of object detection and tracking algorithm for body pose recognition.
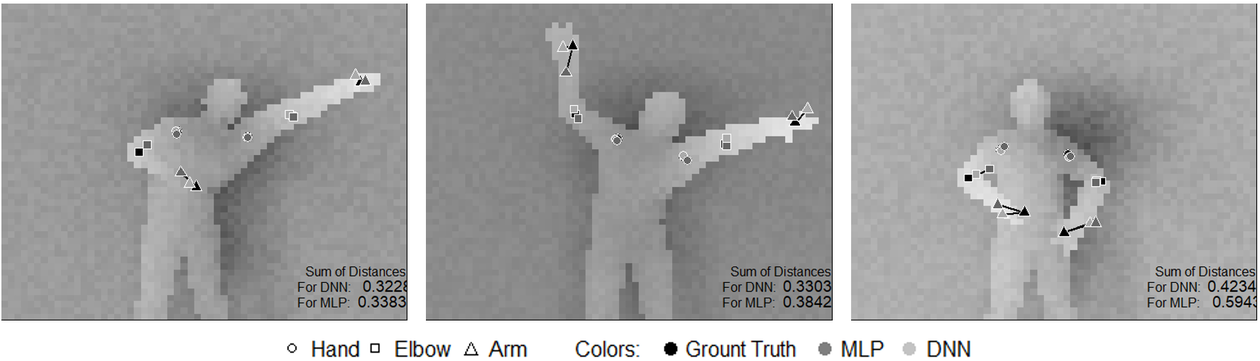
Dataset file
hexmd5(md5(part1)+md5(part2)+...)-{parts_count}
where a single part of the file is 512 MB in size.Example script for calculation:
https://github.com/antespi/s3md5
File details
- License:
-
open in new tab
CC BYAttribution
Details
- Year of publication:
- 2020
- Verification date:
- 2020-12-17
- Dataset language:
- English
- Fields of science:
-
- information and communication technology (Engineering and Technology)
- DOI:
- DOI ID 10.34808/84xp-vz47 open in new tab
- Verified by:
- Gdańsk University of Technology
Keywords
References
- publication ANN for human pose estimation in low resolution depth images
- publication CNN Architectures for Human Pose Estimation from a Very Low Resolution Depth Image
- publication Deep neural networks for human pose estimation from a very low resolution depth image
Cite as
Authors
seen 297 times