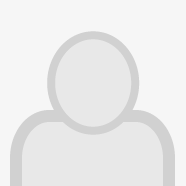
dr inż. Sebastian Cygert
Employment
- IT Specialist at Department of Multimedia Systems
- Assistant professor at Department of Multimedia Systems
Publications
Filters
total: 36
Catalog Publications
Year 2024
-
Adapt Your Teacher: Improving Knowledge Distillation for Exemplar-free Continual Learning
PublicationIn this work, we investigate exemplar-free class incremental learning (CIL) with knowledge distillation (KD) as a regularization strategy, aiming to prevent forgetting. KDbased methods are successfully used in CIL, but they often struggle to regularize the model without access to exemplars of the training data from previous tasks. Our analysis reveals that this issue originates from substantial representation shifts in the teacher...
-
Category Adaptation Meets Projected Distillation in Generalized Continual Category Discovery
Publication"Generalized Continual Category Discovery (GCCD) tackles learning from sequentially arriving, partially labeled datasets while uncovering new categories. Traditional methods depend on feature distillation to prevent forgetting the old knowledge. However, this strategy restricts the model’s ability to adapt and effectively distinguish new categories. To address this, we introduce a novel technique integrating a learnable projector...
-
Deep Learning-Based, Multiclass Approach to Cancer Classification on Liquid Biopsy Data
Publication -
Deep Learning-Based, Multiclass Approach to Cancer Classification on Liquid Biopsy Data
PublicationThe field of cancer diagnostics has been revolutionized by liquid biopsies, which offer a bridge between laboratory research and clinical settings. These tests are less invasive than traditional biopsies and more convenient than routine imaging methods. Liquid biopsies allow studying of tumor-derived markers in bodily fluids, enabling the development of more precise cancer diagnostic tests for screening, disease monitoring, and...
-
Divide and not forget: Ensemble of selectively trained experts in Continual Learning
PublicationClass-incremental learning is becoming more popular as it helps models widen their applicability while not forgetting what they already know. A trend in this area is to use a mixture-of-expert technique, where different models work together to solve the task. However, the experts are usually trained all at once using whole task data, which makes them all prone to forgetting and increasing computational burden. To address this limitation,...
-
Improving platelet‐RNA‐based diagnostics: a comparative analysis of machine learning models for cancer detection and multiclass classification
PublicationLiquid biopsy demonstrates excellent potential in patient management by providing a minimally invasive and cost-effective approach to detecting and monitoring cancer, even at its early stages. Due to the complexity of liquid biopsy data, machine-learning techniques are increasingly gaining attention in sample analysis, especially for multidimensional data such as RNA expression profiles. Yet, there is no agreement in the community...
-
Looking through the past: better knowledge retention for generative replay in continual learning
PublicationIn this work, we improve the generative replay in a continual learning setting to perform well on challenging scenarios. Because of the growing complexity of continual learning tasks, it is becoming more popular, to apply the generative replay technique in the feature space instead of image space. Nevertheless, such an approach does not come without limitations. In particular, we notice the degradation of the continually trained...
-
MagMax: Leveraging Model Merging for Seamless Continual Learning
PublicationThis paper introduces a continual learning approach named MagMax, which utilizes model merging to enable large pre-trained models to continuously learn from new data without forgetting previously acquired knowledge. Distinct from traditional continual learning methods that aim to reduce forgetting during task training, MagMax combines sequential fine-tuning with a maximum magnitude weight selection for effective knowledge integration...
-
Revisiting Supervision for Continual Representation Learning
Publication"In the field of continual learning, models are designed to learn tasks one after the other. While most research has centered on supervised continual learning, there is a growing interest in unsupervised continual learning, which makes use of the vast amounts of unlabeled data. Recent studies have highlighted the strengths of unsupervised methods, particularly self-supervised learning, in providing robust representations. The improved...
-
Task-recency bias strikes back: Adapting covariances in Exemplar-Free Class Incremental Learning
PublicationExemplar-Free Class Incremental Learning (EFCIL) tackles the problem of training a model on a sequence of tasks without access to past data. Existing state-of-the-art methods represent classes as Gaussian distributions in the feature extractor's latent space, enabling Bayes classification or training the classifier by replaying pseudo features. However, we identify two critical issues that compromise their efficacy when the feature...
Year 2023
-
Cross-Lingual Knowledge Distillation via Flow-Based Voice Conversion for Robust Polyglot Text-to-Speech
PublicationIn this work, we introduce a framework for cross-lingual speech synthesis, which involves an upstream Voice Conversion (VC) model and a downstream Text-To-Speech (TTS) model. The proposed framework consists of 4 stages. In the first two stages, we use a VC model to convert utterances in the target locale to the voice of the target speaker. In the third stage, the converted data is combined with the linguistic features and durations...
-
Platelet RNA Sequencing Data Through the Lens of Machine Learning
PublicationLiquid biopsies offer minimally invasive diagnosis and monitoring of cancer disease. This biosource is often analyzed using sequencing, which generates highly complex data that can be used using machine learning tools. Nevertheless, validating the clinical applications of such methods is challenging. It requires: (a) using data from many patients; (b) verifying potential bias concerning sample collection; and (c) adding interpretability...
Year 2022
-
Creating new voices using normalizing flows
PublicationCreating realistic and natural-sounding synthetic speech remains a big challenge for voice identities unseen during training. As there is growing interest in synthesizing voices of new speakers, here we investigate the ability of normalizing flows in text-to-speech (TTS) and voice conversion (VC) modes to extrapolate from speakers observed during training to create unseen speaker identities. Firstly, we create an approach for TTS...
-
Robust and Efficient Machine Learning Algorithms for Visual Recognition
PublicationIn visual recognition, the task is to identify and localize all objects of interest in the input image. With the ubiquitous presence of visual data in modern days, the role of object recognition algorithms is becoming more significant than ever and ranges from autonomous driving to computer-aided diagnosis in medicine. Current models for visual recognition are dominated by models based on Convolutional Neural Networks (CNNs), which...
-
Robust Object Detection with Multi-input Multi-output Faster R-CNN
PublicationRecent years have seen impressive progress in visual recognition on many benchmarks, however, generalization to the out-of-distribution setting remains a significant challenge. A state-of-the-art method for robust visual recognition is model ensembling. However, recently it was shown that similarly competitive results could be achieved with a much smaller cost, by using multi-input multi-output architecture (MIMO). In this work,...
-
Robust Object Detection with Multi-input Multi-output Faster R-CNN
PublicationRecent years have seen impressive progress in visual recognition on many benchmarks, however, generalization to the out-of-distribution setting remains a significant challenge. A state-of-the-art method for robust visual recognition is model ensembling. However, recently it was shown that similarly competitive results could be achieved with a much smaller cost, by using multi-input multi-output architecture (MIMO). In this work,...
Year 2021
-
Closer Look at the Uncertainty Estimation in Semantic Segmentation under Distributional Shift
PublicationWhile recent computer vision algorithms achieve impressive performance on many benchmarks, they lack robustness - presented with an image from a different distribution, (e.g. weather or lighting conditions not considered during training), they may produce an erroneous prediction. Therefore, it is desired that such a model will be able to reliably predict its confidence measure. In this work, uncertainty estimation for the task...
-
Robustness in Compressed Neural Networks for Object Detection
PublicationModel compression techniques allow to significantly reduce the computational cost associated with data processing by deep neural networks with only a minor decrease in average accuracy. Simultaneously, reducing the model size may have a large effect on noisy cases or objects belonging to less frequent classes. It is a crucial problem from the perspective of the models' safety, especially for object detection in the autonomous driving...
-
Towards Cancer Patients Classification Using Liquid Biopsy
PublicationLiquid biopsy is a useful, minimally invasive diagnostic and monitoring tool for cancer disease. Yet, developing accurate methods, given the potentially large number of input features, and usually small datasets size remains very challenging. Recently, a novel feature parameterization based on the RNA-sequenced platelet data which uses the biological knowledge from the Kyoto Encyclopedia of Genes and Genomes, combined with a classifier...
Year 2020
-
Evaluating calibration and robustness of pedestrian detectors
PublicationIn this work robustness and calibration of modern pedestrian detectors are evaluated. Pedestrian detection is a crucial perception com- ponent in autonomous driving and here we study its performance under different image corruptions. Furthermore, we provide analysis of classifi- cation calibration of pedestrian detectors and we show a positive effect of using style-transfer augmentation technique. Our analysis is aimed as a step...
-
Evaluating Calibration and Robustness of Pedestrian Detectors
Publication -
Toward Robust Pedestrian Detection With Data Augmentation
PublicationIn this article, the problem of creating a safe pedestrian detection model that can operate in the real world is tackled. While recent advances have led to significantly improved detection accuracy on various benchmarks, existing deep learning models are vulnerable to invisible to the human eye changes in the input image which raises concerns about its safety. A popular and simple technique for improving robustness is using data...
-
Vehicle Detection with Self-Training for Adaptative Video Processing Embedded Platform
PublicationTraffic monitoring from closed-circuit television (CCTV) cameras on embedded systems is the subject of the performed experiments. Solving this problem encounters difficulties related to the hardware limitations, and possible camera placement in various positions which affects the system performance. To satisfy the hardware requirements, vehicle detection is performed using a lightweight Convolutional Neural Network (CNN), named...
Year 2019
-
Comparative study on the effectiveness of various types of road traffic intensity detectors
PublicationVehicle detection and speed measurements are crucial tasks in traffic monitoring systems. In this work, we focus on several types of electronic sensors, operating on different physical principles in order to compare their effectiveness in real traffic conditions. Commercial solutions are based on road tubes, microwave sensors, LiDARs, and video cameras. Distributed traffic monitoring systems require a high number of monitoring...
-
Recovering Sound Produced by Wind Turbine Structures Employing Video Motion Magnification
PublicationThe recordings were made with a fast video camera and with a microphone. Using fast cameras allowed for observation of the micro vibrations of the object structure. Motion-magnified video recordings of wind turbines on a wind farm were made for the purpose of building a damage prediction system. An idea was to use video to recover sound & vibrations in order to obtain a contactless diagnostic method for wind turbines. The recovered signals...
-
Style Transfer for Detecting Vehicles with Thermal Camera
Publication -
Style Transfer for Detecting Vehicles with Thermal Camera
PublicationIn this work we focus on nighttime vehicle detection for intelligent traffic monitoring from the thermal camera. To train a Convolutional Neural Network (CNN) detector we create a stylized version of COCO (Common Objects in Context) dataset using Style Transfer technique that imitates images obtained from thermal cameras. This new dataset is further used for fine-tuning of the model and as a result detection accuracy on images...
-
Vehicle detector training with minimal supervision
PublicationRecently many efficient object detectors based on convolutional neural networks (CNN) have been developed and they achieved impressive performance on many computer vision tasks. However, in order to achieve practical results, CNNs require really large annotated datasets for training. While many such databases are available, many of them can only be used for research purposes. Also some problems exist where such datasets are not...
-
Wind Turbines Modeling as the Tool for Developing Algorithms of Processing their Video Recordings
PublicationIn the real world, many factors exist disturbing observation of the examined phenomena and causing various noises and distortions in recorded signals. It very often makes it difficult or even impossible to optimize various signal processing algorithms, through finding appropriate parameters. In this paper, we show an application, that retrieves wind turbine rotor speed from recorded video. Next, we describe the process of reduction...
-
Wind Turbines Modeling as the Tool for Developing Algorithms of Processing their Video Recordings
PublicationIn the real world, many factors exist disturbing observation of the examined phenomena and causing various noises and distortions in recorded signals. It very often makes it difficult or even impossible to optimize various signal processing algorithms, through finding appropriate parameters. In this paper, we show an application, that retrieves wind turbine rotor speed from recorded video. Next, we describe the process of reduction...
Year 2018
-
Eulerian motion magnification applied to structural health monitoring of wind turbines
PublicationSeveral types of defects may occur in wind turbines, as physical damage of blades or gearbox malfunction. A wind farm monitoring and damage prediction system is built to observe abnormal vibrations of elements of wind turbine: blades, nacelle, and tower. Contactless methods are developed which do not require turbine stopping. In this work, structural health monitoring of a wind turbine is evaluated using a conversion from the captured...
-
REJESTRACJA, PARAMETRYZACJA I KLASYFIKACJA ALOFONÓW Z WYKORZYSTANIEM BIMODALNOŚCI
PublicationPraca dotyczy rejestracji i parametryzacji alofonów w języku angielskim z wykorzystaniem dwóch modalności. W badaniach dokonano rejestracji wypowiedzi w języku angielskim mówców, których znajomość tego języka odpowiada poziomowi rodowitego mówcy. W kolejnym etapie wyodrębnione zostały alofony z nagrań fonicznych i odpowiadające im sygnały wizyjne. W procesie tworzenia wektorów cech wykorzystano odrębne systemy parametryzacji,...
-
Vehicle detector training with labels derived from background subtraction algorithms in video surveillance
PublicationVehicle detection in video from a miniature station- ary closed-circuit television (CCTV) camera is discussed in the paper. The camera provides one of components of the intelligent road sign developed in the project concerning the traffic control with the use of autonomous devices being developed. Modern Convolutional Neural Network (CNN) based detectors need big data input, usually demanding their manual labeling. In the presented...
-
Vocalic Segments Classification Assisted by Mouth Motion Capture
PublicationVisual features convey important information for automatic speech recognition (ASR), especially in noisy environment. The purpose of this study is to evaluate to what extent visual data (i.e. lip reading) can enhance recognition accuracy in the multi-modal approach. For that purpose motion capture markers were placed on speakers' faces to obtain lips tracking data during speaking. Different parameterizations strategies were tested...
Year 2017
-
Shape-Based Pose Estimation of Robotic Surgical Instruments
PublicationWe describe a detector of robotic instrument parts in image-guided surgery. The detector consists of a huge ensemble of scale-variant and pose-dedicated, rigid appearance templates. The templates, which are equipped with pose-related keypoints and segmentation masks, allow for explicit pose estimation and segmentation of multiple end-effectors as well as fine-grained non-maximum suppression. We train the templates by grouping examples...
Year 2014
seen 2003 times